A key reason for these shortcomings is that claims and hospital chargemaster data are defined by payer or reimbursement requirements. Since payers don’t require detailed device data, neither medical claims nor chargemaster data tend to include it. This severely limits the ability to carry out device research that requires an understanding of the specific clinical context and outcomes linked to device use.
How EHR data can fill critical evidence gaps
Designed to document treatment and care interactions in detail, data from EHRs can offer deep clinical insights about patient health not present in claims-related data, such as lab test results, clinical outcomes, symptoms, and side effects. Additional information on medical device use and outcomes is often found as semi-structured data (text strings in fields) or free text in clinician notes that are not captured elsewhere. These clinical details are vital to understanding the overall patient journey.
To better illustrate this, let’s consider the following patient story:
-
- A patient receives a pacemaker at a hospital.
- The medical billing team adds the relevant CPT code to the chargemaster for the patient with the descriptor for “Insertion of new or replacement of permanent pacemaker with transvenous electrode(s); atrial and ventricular.”
- The relevant CPT codes and procedure data are also captured in the EHR, with the patient’s care team adding notes on device manufacturer and brand and clinical observations.
- 4 years later, the patient has the pacemaker replaced. Since CPT codes are standardized, the medical billing team adds the same billing code to the chargemaster. However, only the EHR contains insight into the reason for the replacement, procedural documentation, and new device information.
In this case, considering the hospital chargemaster data alone may not provide any information on the specific device brand or clinical observations related to the reason for replacement. Though researchers may be able to use proxy information to determine device make and model in some instances, research used to inform key decisions requires stronger evidence. The patient’s EHR records, including procedure data from chargemasters, can provide the required clinical evidence necessary for comparative effectiveness studies and safety monitoring.
However, the potential of EHR data for clinical research on medical devices has historically not been realized for several reasons: 1) EHR data, while clinically rich, is often difficult to access due to patient privacy concerns and regulations. 2) EHR data is hard to analyze: it is incredibly fragmented and unstructured, especially when aggregated across many healthcare organizations. 3) Valuable information is often hidden in clinician notes. A researcher is more likely to find vital details about outcomes and clinical measurement in free-text clinical notes than structured fields.
How Truveta creates clean and complete medical device data from EHRs
Truveta captures full medical records for patients from a growing collective of more than 30 major health systems, representing over 17% of care in the US.
The data captured during treatment interactions by busy clinicians is not organized and is hard to analyze, particularly when aggregated across EHRs from thousands of sites of care using different systems. There are billions of clinical concepts for diagnoses, devices, procedures, etc. which can be expressed in a number of ways – especially when you consider misspellings and abbreviations.
To address this, Truveta created Truveta Language Model (TLM), a large language, multi-modal AI model used to clean billions of daily EHR data points for health research. TLM’s healthcare expertise is trained on the most complete collection of complete medical records, representing the full diversity of the United States.
TLM is constantly reviewed and trained by clinical experts on an unprecedented scale of EHR data to drive high clinical accuracy, without the commercial bias driven by reimbursement often found in claims data.
In the case of medical devices, TLM standardizes device information to the Truveta device hierarchy, which includes the company- and brand-specific information necessary for research that standard terminologies often lack. The device hierarchy is based on three fields provided in the Global Unique Device Identification Database (GUDID): company name, brand name, and product code name; with the unique device identifier (UDI) associated when available.
Consider the example below: for just one device, Mitraclip, we might see many variations in EHR data that need to be standardized to make the information useful for analysis. TLM maps the various descriptions for Mitraclip to a standardized company, brand, and product type, as well as the UDI where available.
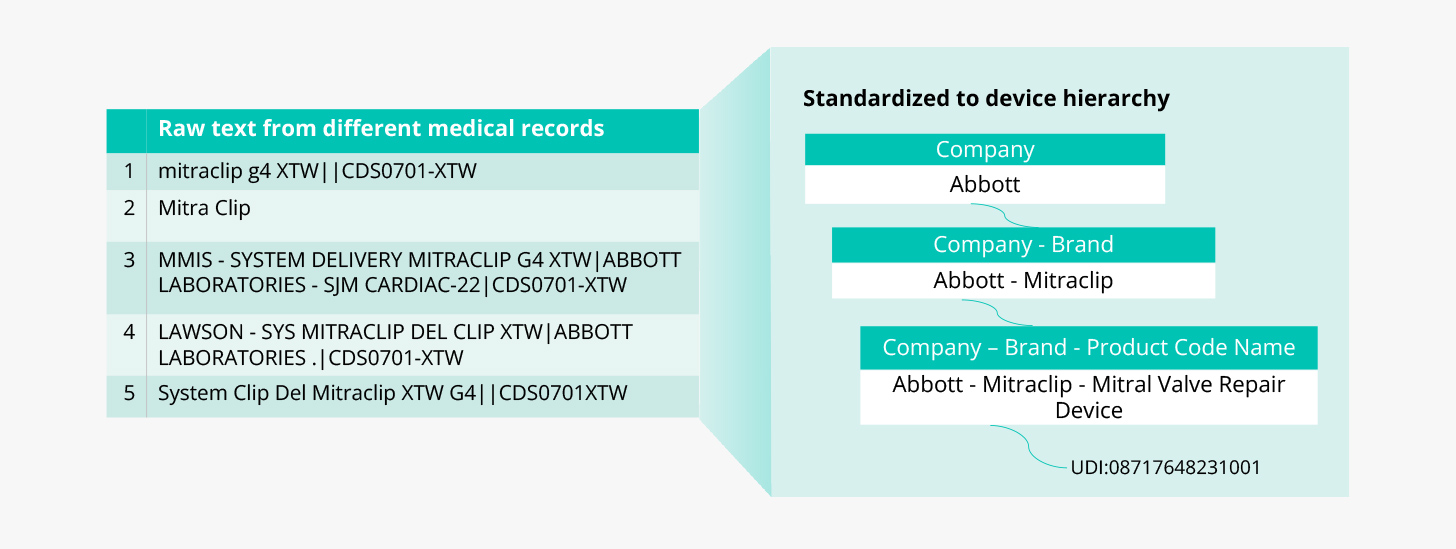
Unlocking additional data contained in clinician notes
Key drug and medical device information is often found in free text notes along with rich clinical details about the patient journey, such as disease signs and symptoms, patient-reported outcomes, stages of disease, and clinical measurements. These concepts are not found in claims, chargemaster, or most structured EHR data. To unlock this information for analytics, TLM combines general large language models that understand English with rich medical expertise to structure concepts from clinician notes.
For example, the redacted clinician note below contains information about a de-identified patient with heart failure. The text contains critical information about the diagnosis, ECHO results, staging of heart failure (NYHA classification), and clinical measurement of ejection fraction (EF).
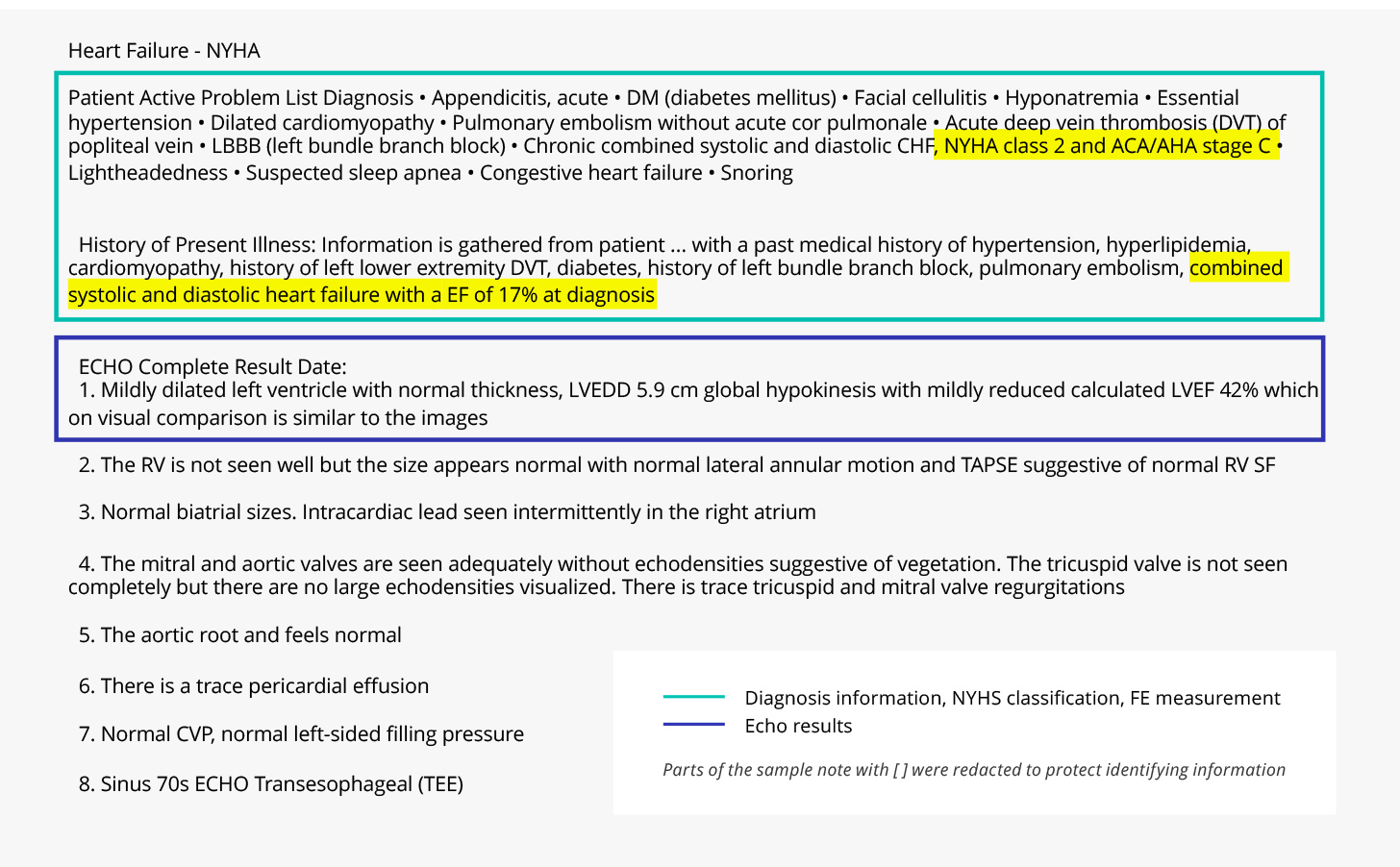
The detailed medical device information and rich clinical context in Truveta Data can transform device R&D to enhance device safety and outcomes, improve patient care, and reduce healthcare costs.
Learn more in this whitepaper, or contact us to discover why Truveta is trusted by more than 40 leading healthcare and life science organizations for fast insights that improve patient care and accelerate R&D.