UPDATE: Truveta has won the 2024 South by Southwest (SXSW) Innovation Award in Artificial Intelligence for its AI-enabled health research and the Truveta Language Model (TLM).
Healthcare data traditionally has been siloed, inaccessible, and too messy to be useful for healthcare research. Truveta focuses on solving all three of these problems. The power of AI allows us to take the messy data and beautifully clean it for analytics.
Today we are excited to introduce the Truveta Language Model (TLM), a large-language, multi-modal AI model for transforming electronic health record (EHR) data into billions of clean and accurate data points for health research on patient outcomes with any drug, disease, or device. TLM’s healthcare expertise is trained on the largest collection of complete medical records representing the full diversity of the United States. It is the first large-language model specifically designed to empower researchers to accurately study patient care and outcomes.
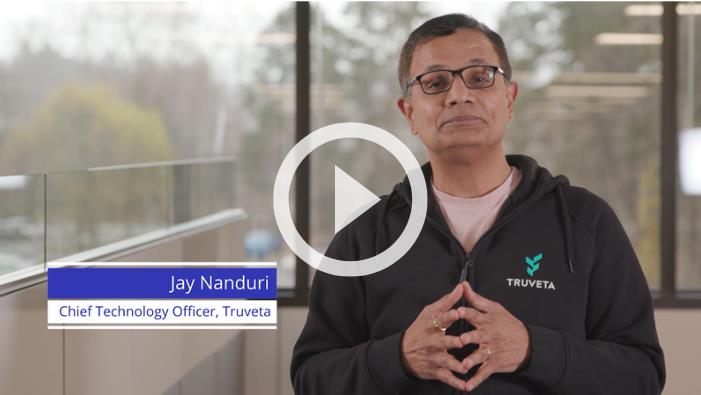
As healthcare considers the potential of AI and real-world data, the opportunities and potential consequences are real. General large language models understand language but are inaccurate within the medical domain due to being trained on the public internet, which contains no real medical records. In contrast, TLM combines pre-trained open large language models with deep training on the most complete and representative clinical data set to achieve above 90% accuracy on diagnoses, medications, lab results, lab values, clinical observations, and more, exceeding the accuracy of human clinical experts.
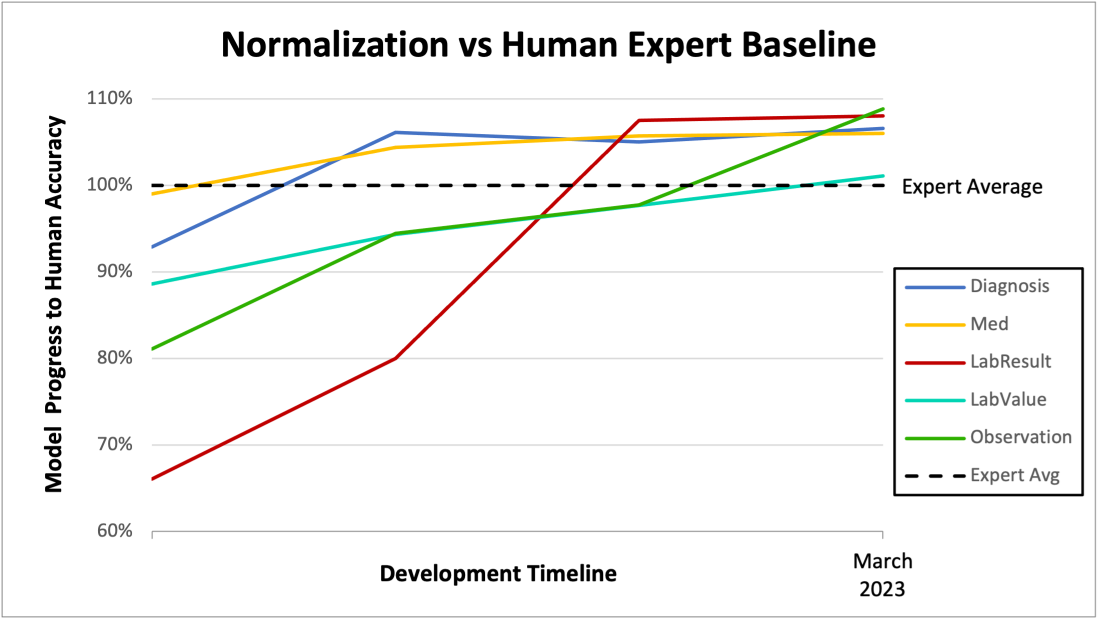
While claims data are the standard of data used in health research today, they are created by normalizing EHR data to maximize revenue reimbursement for encounters, medications, and labs, resulting in commercial bias in all claims data-based health research. Instead, TLM normalizes EHR data to maximize clinical accuracy and is trained without commercial bias, helping ensure research is conducted with data focused on clinical outcomes, not billing.
With TLM, Truveta’s community of healthcare and life science customers are currently studying concepts previously inaccessible in messy clinician notes but now structured for analytics, such as seizure frequency, changes in treatment regimen, and adverse reactions to medication.
By using clinical expert-led AI to unlock the power of rich healthcare data, researchers can now ask and answer complex medical questions of a real-time, fully transparent view of U.S. health.
Delivering the cleanest healthcare data
Healthcare data is recorded in heterogeneous systems with millions of different ways clinicians, hospitals, and health systems express observations, diagnoses, medication plans, and more. Clinicians use different terms based on their location, training, and expertise. “Acute COVID-19,” “COVID,” “COVID-19,” “COVID infection,” and “COVID19 _ acute infection” (and hundreds of other variations) all refer to COVID-19, and “600mg Ibuprofen” and “Ibuprofen 600mg” are the same thing. To analyze healthcare data, this diverse medical language, including misspellings or abbreviations, must be normalized to medical information ontologies (e.g., LOINC for lab tests, GUDID for medical devices, etc.).
AI models are only as good as the data they are trained upon. TLM is trained upon data from Truveta’s health system members currently representing more than 80 million patient journeys, including 5.5 billion diagnoses, 3.1 billion encounters, and 2.4 billion medication orders. Updated daily, Truveta Data combines this EHR data with social drivers of health (SDOH) data, claims, and mortality for unmatched breadth and depth of data for research. Using this unprecedented data, Truveta’s clinical expert annotators label tens of thousands of raw clinical terms to train TLM to normalize healthcare data for clinical accuracy, and then check the results of the model as it runs.
TLM accurately, without commercial bias, cleans the complete EHR medical record for analytics. For example, consider two blood lab tests that TLM structures into four rows of the LabResults table within our Truveta Data Model. Each test is mapped to a standard medical ontology with standard units for the measurements.
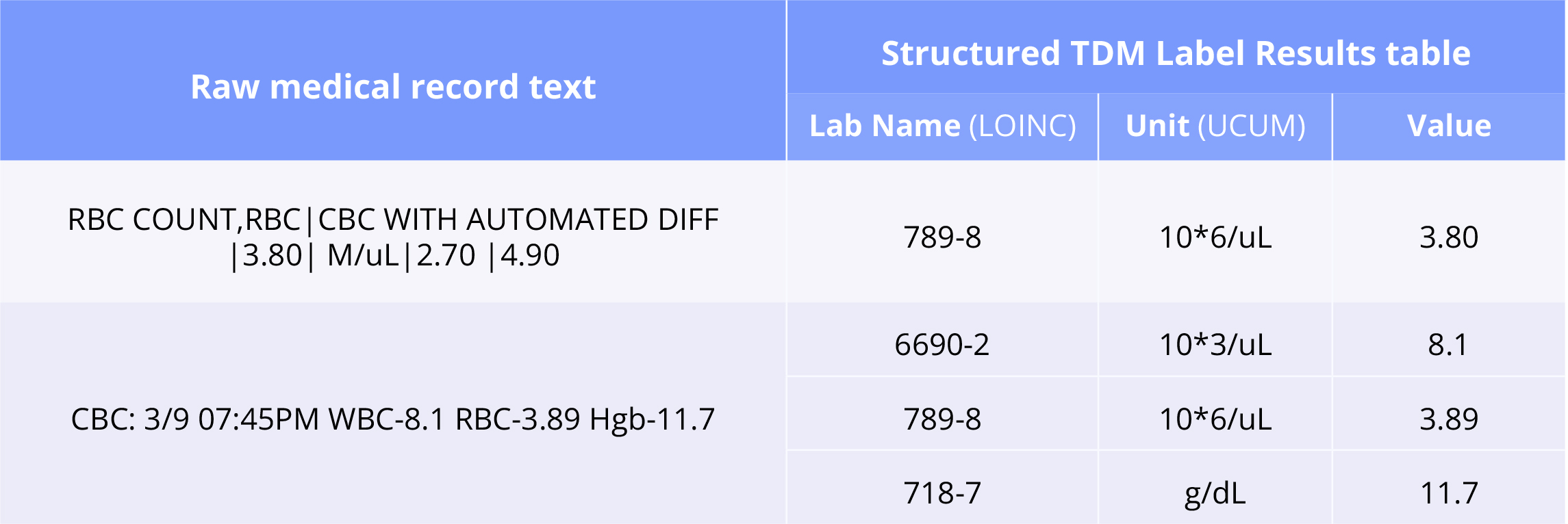
You can read even more about the process of normalization using TLM, in the Truveta Language Model whitepaper.
Clinical accuracy vs GPT-4
The results of this training and expert guiding process are accuracy in understanding medical records. By comparison GPT-4, which does not train on real medical records, has been found to hallucinate and make up codes that don’t exist, often being completely mistaken (while confidently providing an answer). Here are a few examples, including the potential implication for the patient:
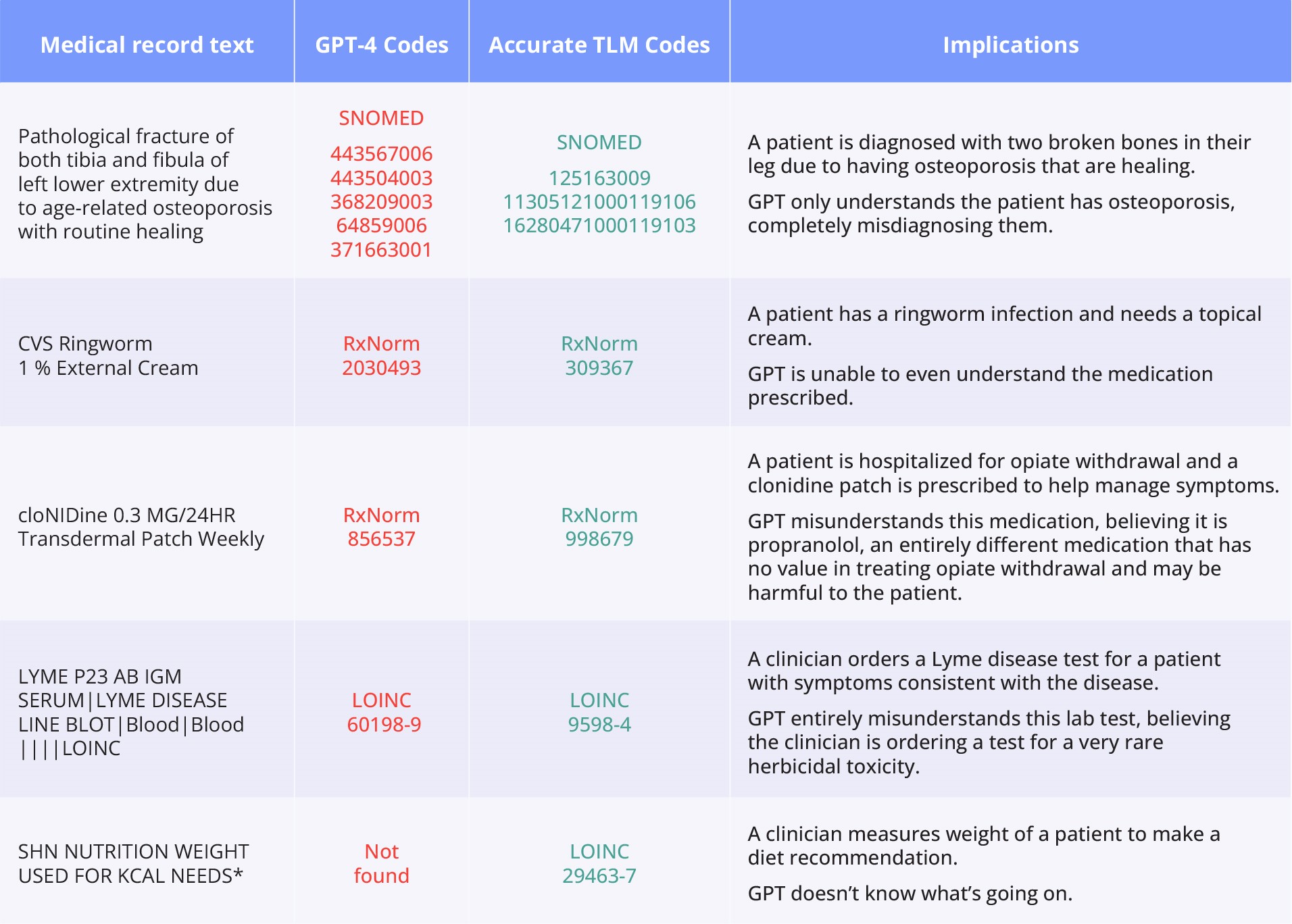
Unlocking the depth of information within clinician notes
Clinician notes hold critical information about the patient journey, such as disease stages, adverse events, medication change rationales, and disease symptoms not found in claims data sets, nor found in most structured EHR analytics data. For example, a structured dataset might include a medication and later a diagnosis of a rash, but the clinician note is the only place where those two concepts are connected, showing the rash as an adverse reaction to the medication.
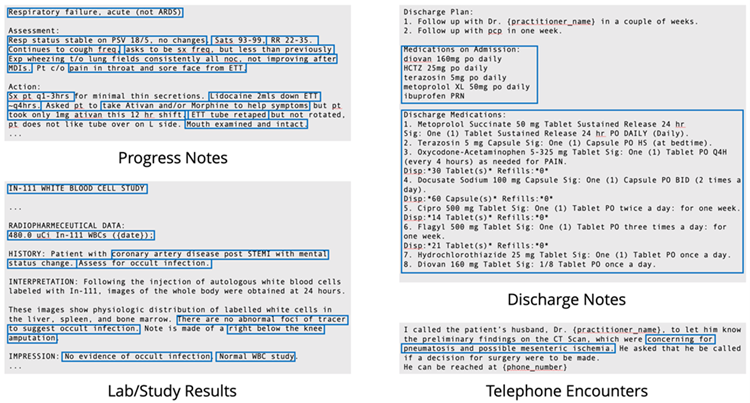
TLM combines general large language models that understand English with rich medical expertise to structure these concepts from clinician notes. Truveta Data today include more than 2.5 billion notes and growing every day. TLM identifies and normalizes clinical concepts within clinician notes, while detecting negation (e.g., “patient denies feeling fatigued”), hypotheticals/conditionals (e.g., “Will consider starting low-dose glipizide if A1C still grossly elevated”), and family history (e.g., “Family Hx: Mother: Diabetes, Father/son: bipolar disorder”).
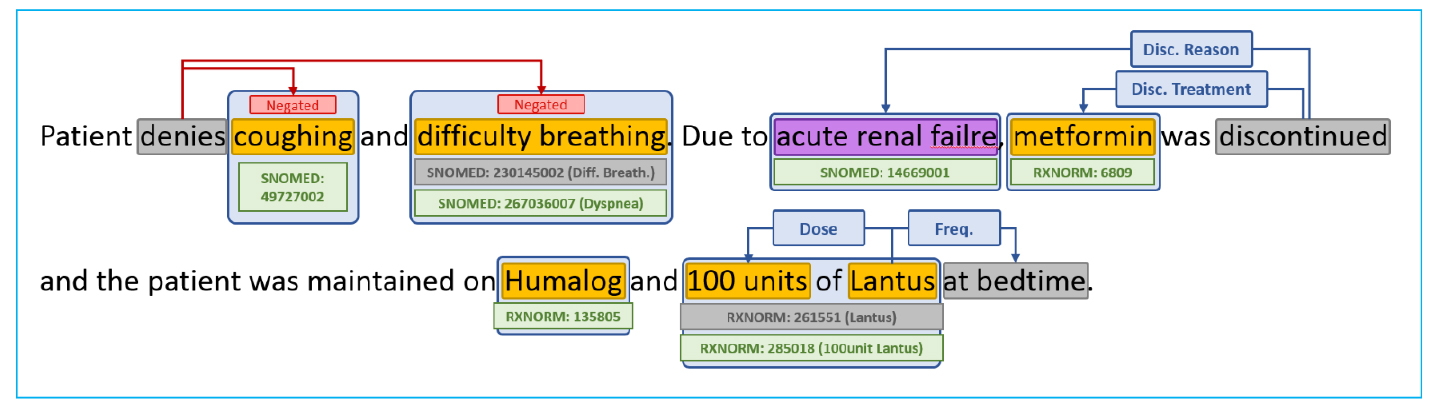
TLM applies reason over the entire medical record, accounting for changes over time, to ensure the most accurate and complete information is structured. With TLM, a researcher studying cancer would be able to see when a therapy is no longer working or when updated images indicate new disease progression that requires a change in treatment.
Advancing clinical research to find cures faster
This massive transformation of healthcare data is only possible with state-of-the-art AI. Truveta’s team of technologists and clinical experts have decades of experience in their domains and are leading the industry in using AI to accurately make health data useful for research. This is one of many AI in healthcare examples.
TLM is a profound innovation for making healthcare data trustworthy and useful for analytics. With Truveta Language Model, Truveta’s community of healthcare and life sciences researchers are studying complete, timely, and clean data to achieve our mission of saving lives with data. We look forward to researchers finding cures faster, empowering every clinician to build expertise, and families being able to make more informed decisions about their care.
Imagine what we can learn together.
— Terry