The Centers for Disease Control and Prevention reports that cardiovascular (CV) disease is the leading cause of death for men, women, and people of most racial and ethnic groups in the US, with 805,000 dying yearly.
Developing improved cardiovascular therapies is costly: According to a 2018 study that included researchers from Johns Hopkins Bloomberg School of Public Health, the mean cost of a CV clinical trial was $157 million, versus just $21 million for pivotal trials in endocrine and metabolic disease patients.
The data dilemma
Real-world data like electronic health records (EHRs), medical claims, chargemasters, and registries have incredible potential to impact R&D efficiency and improve outcomes by providing insights about treatment in the real world. However, while pharma companies have used RWD to generate evidence on the effectiveness and safety of new cardiovascular drugs at a growing rate over the last decade, researchers are often frustrated by data missingness and timeliness. For example, lab values may only be available 30% of the time, leaving critical evidence gaps for research, and many RWD providers offer data that is 6-12 months old.
For device manufacturers, studying patient outcomes is particularly challenging: there is a significant lack of detailed device data in RWD sources. Claims and chargemaster data do not include information about device manufacturers and brands, clinical outcomes, or lab values, as they are designed for insurance reimbursement and revenue management, not patient care.
EHR data offers the clinical depth missing in other RWD sources, including specific device detail, and presents excellent potential for CV research. Still, its use has been limited, as EHR data aggregated from various sites of care are fragmented, not standardized and difficult to analyze at scale. At the same time, critical clinical information is present across a mix of semi-structured and unstructured (free text clinical notes).
Creating complete and clean EHR data with clinical expert-led AI
Truveta, a leader in EHR data and analytics, led by a growing health system collective providing more than 17% of all daily clinical care in the US, has harnessed the power of artificial intelligence to overcome these data challenges. At the heart of Truveta’s approach to creating clean and complete EHR data at scale is the Truveta Language Model (TLM), a large-language AI model that is trained on the largest collection of complete medical records representing the full diversity of the United States. It is the first large-language model specifically designed for researchers to accurately study patient care and outcomes, without the commercial bias found in claims data, which is normalized for revenue optimization.
For example, in the figure below we see six variations of how clinicians might refer to a natriuretic peptide B lab test.
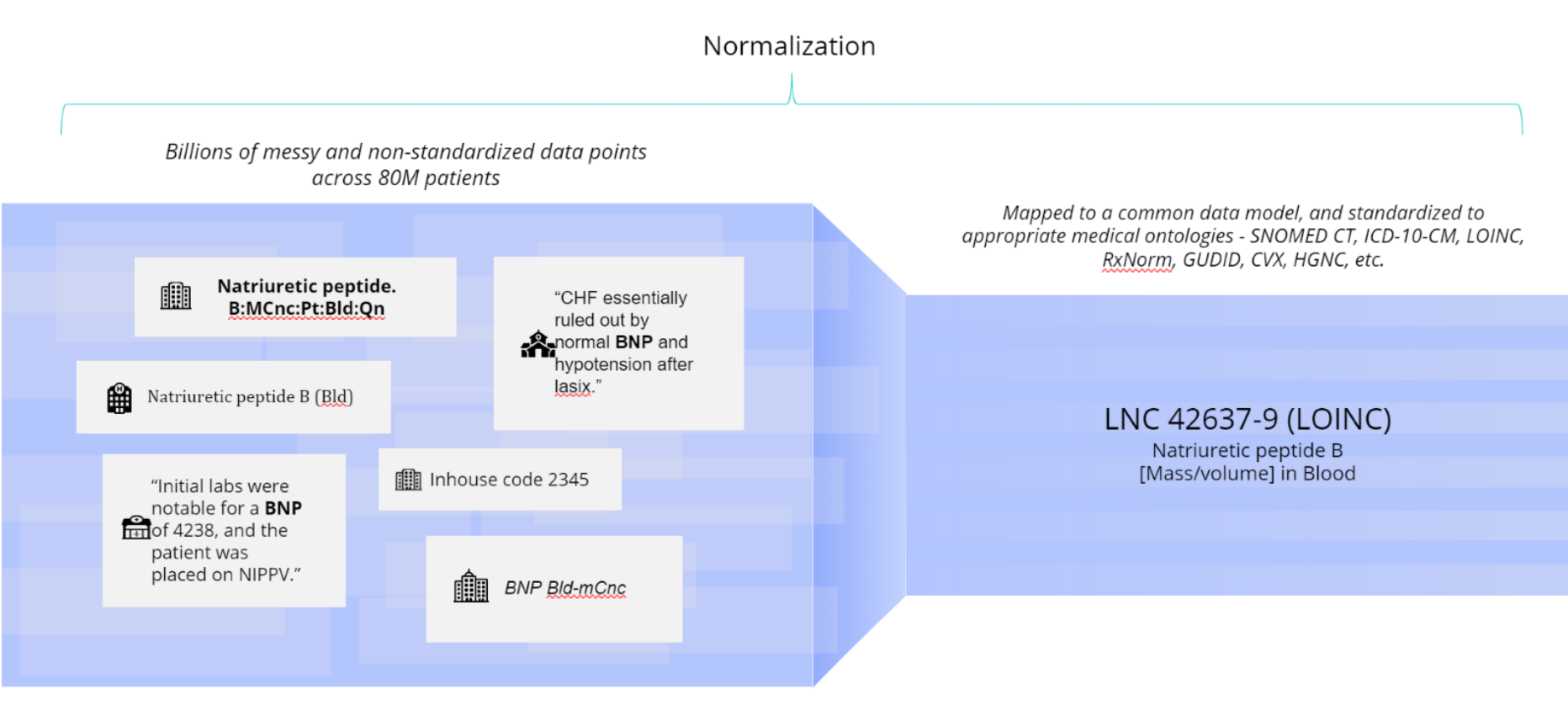
In this case, Truveta Language Model (TLM) standardizes the varying text against the LOINC database. TLM is trained to use the appropriate medical ontology for each clinical concept. TLM is constantly reviewed and trained by clinical experts to drive high clinical accuracy. Truveta’s clinical expert annotation team labels thousands of raw clinical terms, including misspellings and abbreviations, to train TLM to normalize daily updated EHR data into billions of clean and accurate data points for research.
In another example, we see five variations of text that all refer to Mitraclip. Each variation must be standardized by TLM to make the information useful for analysis.
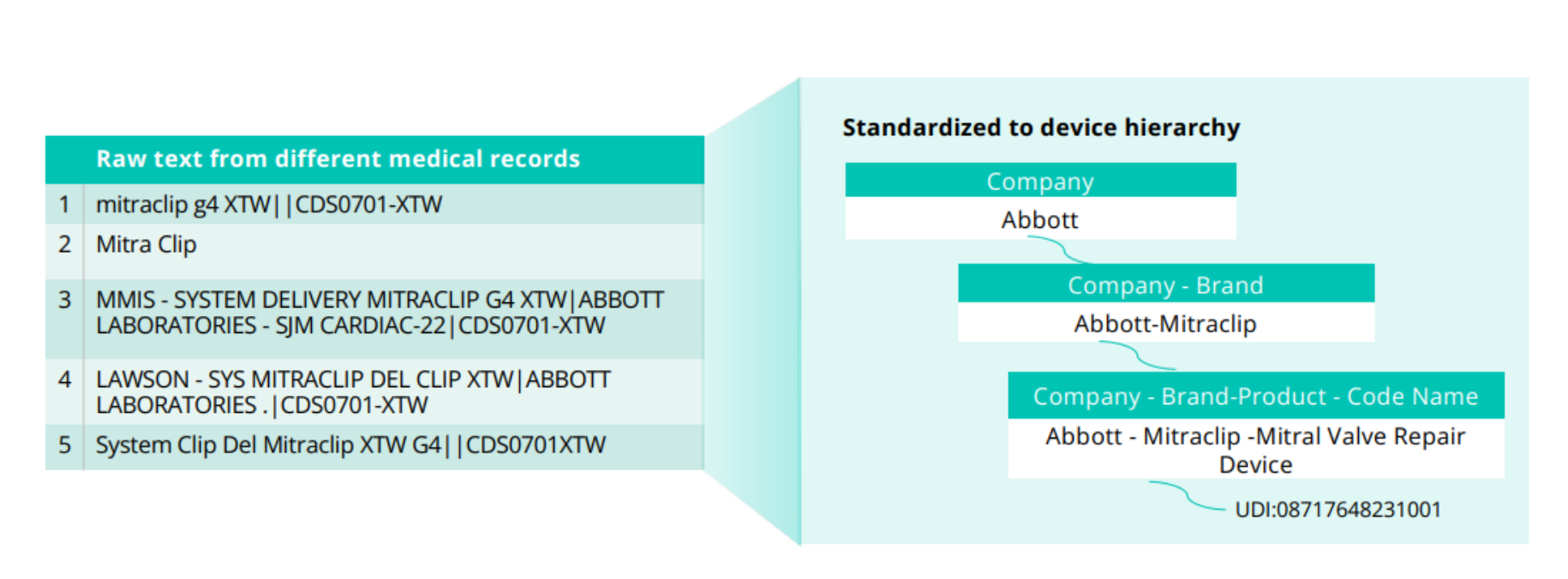
TLM standardizes device information to the Truveta device hierarchy, which includes the company and brand-specific information necessary for research that standard terminologies often lack. The device hierarchy is based on three fields provided in the GUDID: company name, brand name, and product code name (associated with the FDA product code assigned to a specific device), with the unique device identifier (UDI) associated where available.
Unlocking meaningful insights from clinician notes
For the medical devices industry, Truveta Language Model enables the ability to extract clinical concepts from clinician notes. Clinician notes often contain specific device data, including information about the company, brand, and model, which is not available in chargemaster or claims data. Through TLM, device companies gain access to critical drug and device-related insights that can drive innovation and inform product development, ensuring a deeper understanding of device performance and patient outcomes.
Information in clinical notes can provide context on disease symptoms and staging, clinical measurements like ejection fraction, drug or device use details and more.
Conclusion
Truveta offers a unique solution to address the persistent data challenges faced by pharma and medical device companies with the most complete, timely, and clean EHR data across nearly 17M cardiovascular patients. To delve deeper into the capabilities of Truveta Data and its impact on cardiovascular drug and device research, we invite you to download our whitepaper titled “Advancing Cardiovascular Research Through Better Data.”