Data captured in electronic health records (EHRs) can provide rich clinical insights about patient journeys and medical histories, gaps in care, treatment outcomes and effectiveness, and more. However, much of this information lies hidden within free-text clinical notes—rather than structured and standardized data fields. Ejection fraction (EF) is a great example of a “hidden” clinical measure.
EF provides a quantitative measure of the heart’s ability to pump blood throughout the body. Individuals with a low EF have an elevated risk of experiencing complications, including arrhythmias, blood clots, heart valve issues, and even cardiac arrest.
Given its clinical significance, EF is frequently used as an endpoint and as an inclusion/exclusion criterion in clinical trials. Changes in the measure from baseline to a specified follow-up timepoint, for example, can be used to assess the efficacy of medications, medical devices, and surgical procedures.
Obtaining ejection fraction data for real-world studies has been challenging in the past
However, even something as critical as EF has not been readily accessible for use in real-world studies because the results are not uniformly recorded. EF is most frequently assessed via an echocardiogram (echo) – an ultrasound taken of the heart – with the results captured as unstructured or semi-structured data within EHRs or medical imaging databases. The numeric result may be captured within the imaging report, for example, while clinician notes may contain descriptive interpretations of results such as normal/abnormal, low/moderate/severe, or reduced/preserved.
This lack of standardized, accessible data has historically made it difficult to use EF as a research measure in large-scale, real-world studies. Instead, researchers have had to employ strategies such as:
-
- Using registry data, which may not capture the full diversity of patients with a particular disease, since participation is often voluntary or limited to specific geographic areas. There is also usually a lag between data collection and availability.
- Relying on physician referral into clinical studies where EF can be carefully documented, which is time-consuming and limits sample sizes.
- Using claims to identify patients with “reduced EF” (diagnosis of systolic heart failure), without capturing precise, quantitative EF measurements, limiting depth of insight. Claims data also lack timeliness and rely on diagnostic codes which have been shown to not effectively distinguish between patients with reduced EF and preserved EF.
How Truveta extracts EF data for research
To support clinical research in CVD, Truveta extracts clinical concepts at scale from echo reports and clinician notes, which when combined with other data from full medical records in EHRs, enable researchers to study outcomes and treatment effects in larger, more representative populations with a greater degree of precision than the above methods allow.
We do this using the Truveta Language Model (TLM), a large-language, multi-modal AI model trained on the complete medical records of nearly 100 million patients. When TLM achieves greater accuracy than clinical experts in a particular healthcare domain, the model is deployed to start normalizing data.
TLM also builds on top of general large language models to understand clinicians’ notes; identifying and normalizing clinical concepts within free-text notes, while accounting for typos/misspellings, clinical nuances such as negation, hypotheticals/conditionals, and family history. TLM reasons over the entire medical record, accounting for changes over time to ensure the most accurate and complete information is structured.
In figure 1 below, you can see from a redacted clinician note that a patient had 17% EF at the time of heart failure diagnosis and that their most recent echo result showed 42% EF. TLM can extract these specific results across patients with available data, enabling more nuanced cardiovascular research studies.
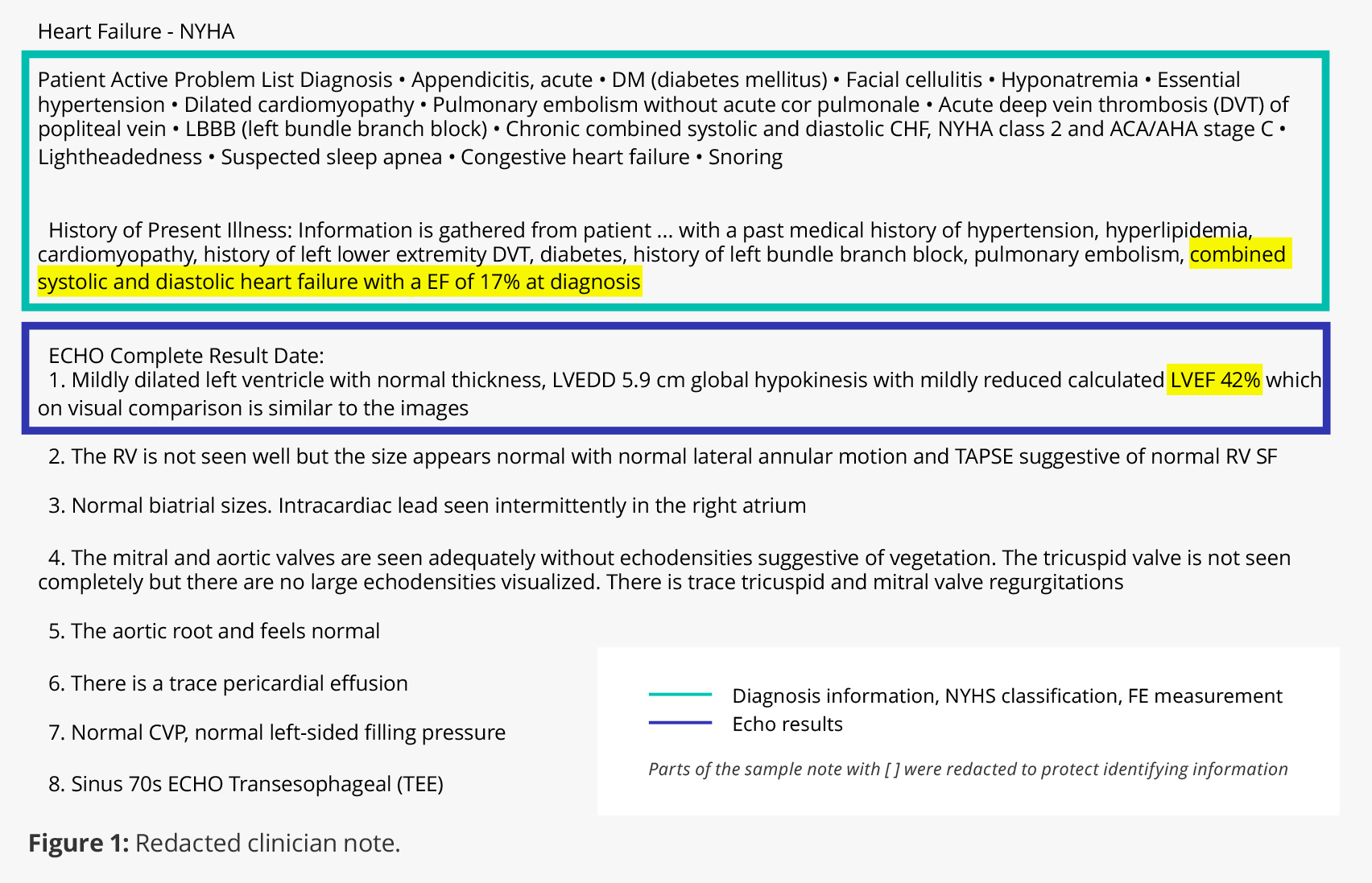
Specific echo report results can also be extracted using the same process. So, rather than only being able to compare patients with “reduced EF” to those with “preserved EF,” for example, researchers can query patients with quantitative EF results (e.g., EF<40%, EF 40%-55%).
Combining ejection fraction data with Truveta’s existing deep EHR data
Truveta Data includes complete EHR data for nearly 100 million patients, including labs, images, and clinician notes. These data are linked across health systems and augmented with social drivers of health and claims data for a complete view of the patient journey. By extracting clinical measures like EF and combining them with other critical data points (updated daily), Truveta offers researchers a much more comprehensive dataset to study:
-
- Treatment effectiveness: By comparing baseline and follow-up EF measures, researchers can assess the impact of medications, devices, cardiac rehabilitation programs, or surgical procedures, and understand the generalizability and applicability of a specific treatment in real-world practice.
- Longitudinal patient outcomes: By tracking changes in EF over time in a specific patient population, researchers can observe disease progression, response to treatment, or natural history of a particular condition.
- Comparative effectiveness: Researchers can compare the impact of different treatment modalities on EF and patient outcomes.
- Disease risk factors: Researchers can investigate the association between risk factors like hypertension, diabetes, and smoking, and reduced EF. This could help identify modifiable risk factors and guide preventive strategies.
- Patient subgroups: Researchers can conduct subgroup analyses based on factors such as age, gender, race, and specific disease etiologies to explore differences in EF data patterns and outcomes within specific patient populations.
Ejection fraction data extraction offers just a single example of the power of the Truveta Language Model. With these capabilities, researchers focusing on cardiovascular disease can also extract other clinical measures from echo reports, such as right ventricle to left ventricle ratio, right ventricle size, and pulmonary artery pressure, as well as related contextual information from clinical notes.
Contact us to learn more about Truveta Data and how concept extraction from clinical notes can help your organization generate more impactful research insights.